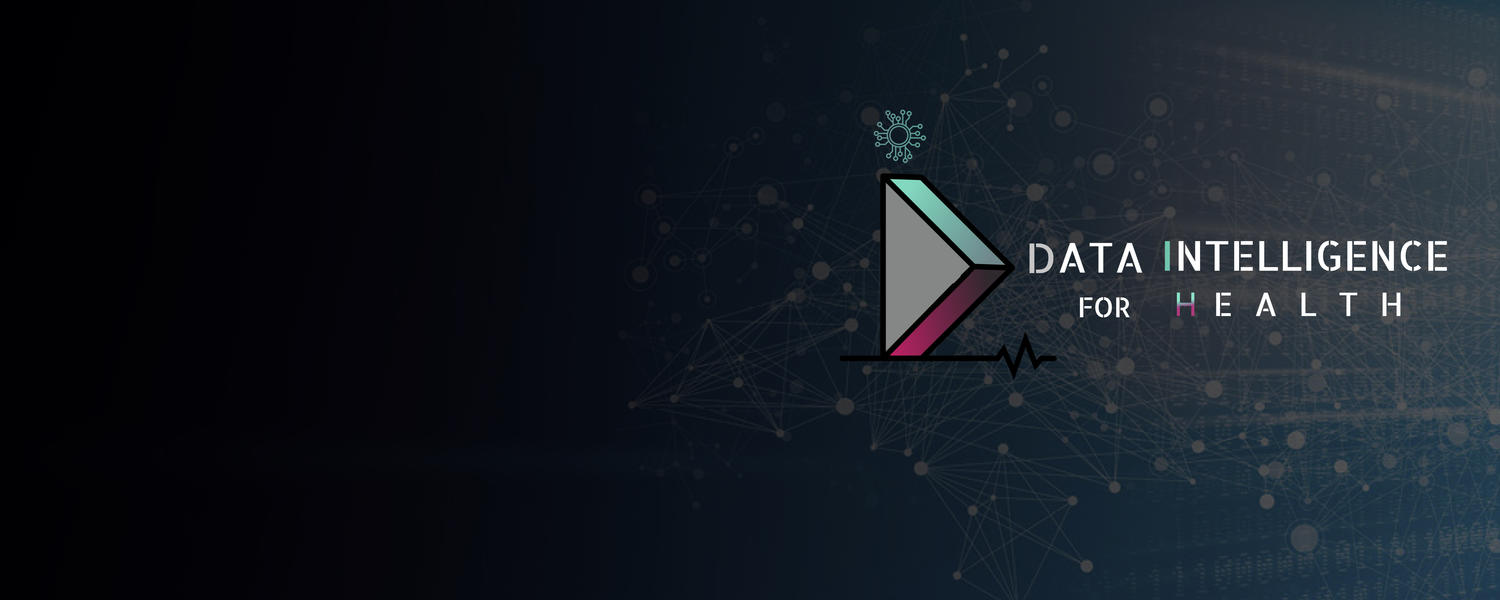
Precision Intensive Care Powered by Data Science and Machine Learning
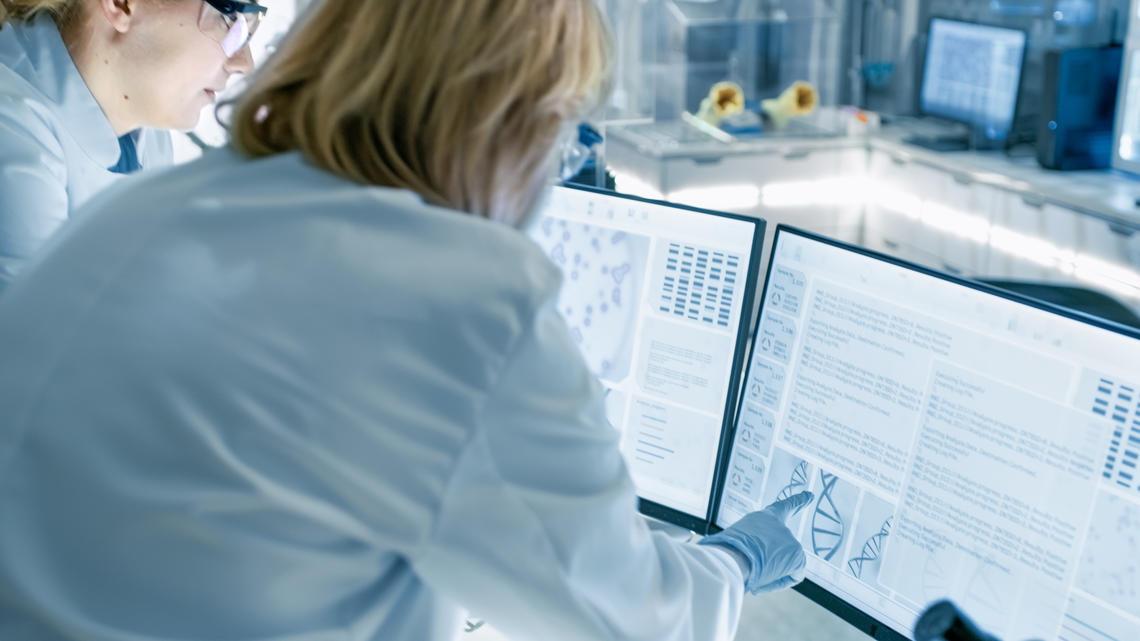
Precision Intensive Care Powered by Data Science and Machine Learning
Intensive care units (ICUs) are an ideal arena for health data science research thanks to their detailed patient monitoring that generates a large volume of rich clinical data. Application of machine learning to ICU data can generate clinically meaningful patient outcome prediction models, leading to early intervention, informed care planning, and efficient resource allocation. We strive to develop and rigorously validate ICU patient outcome prediction models that take into account individual patients’ unique states and characteristics (i.e., precision medicine). Toward this goal, we apply cutting-edge data science and machine learning methods to three large-scale ICU databases: eCritical from Alberta, and public databases called MIMIC and eICU from the United States. Our goals are to predict important ICU outcomes and events (e.g., mortality, sepsis, acute kidney injury, delirium, acute respiratory distress syndrome) in a granular fashion, and to pave ways for our prediction models to be adopted in real-world ICUs. We pay special attention to unconventional sources of predictive information such as missing data or unstructured free-text clinician notes.
Related Publications
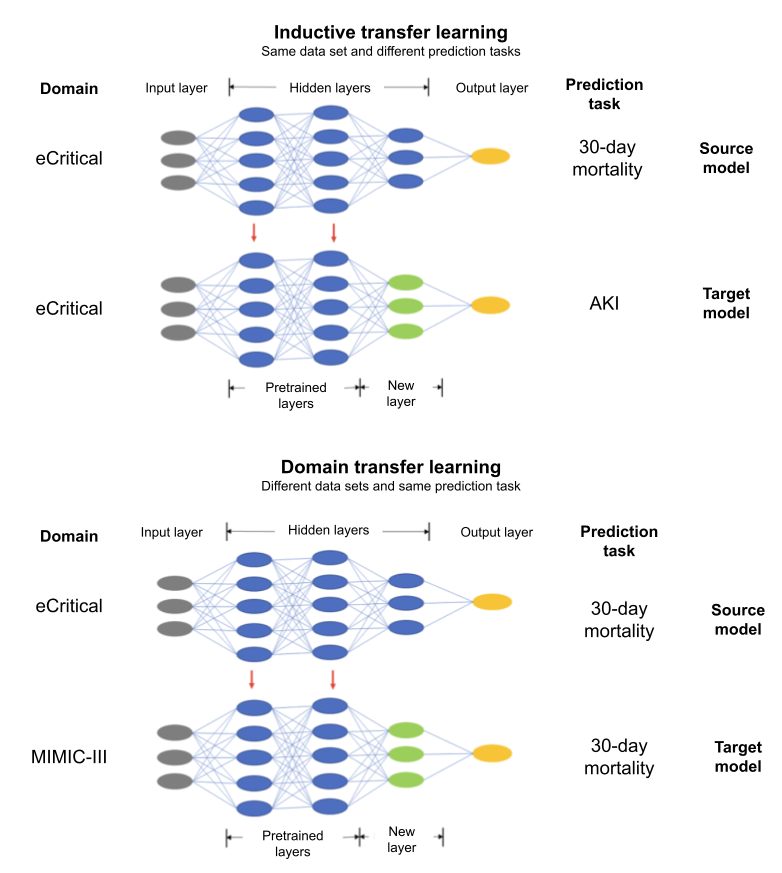
M. K. Mutnuri, H. T. Stelfox, N. D. Forkert, and J. Lee. Using domain adaptation and inductive transfer learning to improve patient outcome prediction in the intensive care unit: retrospective observational study. Journal of Medical Internet Research, 26:e52730, August 2024. [GitHub]
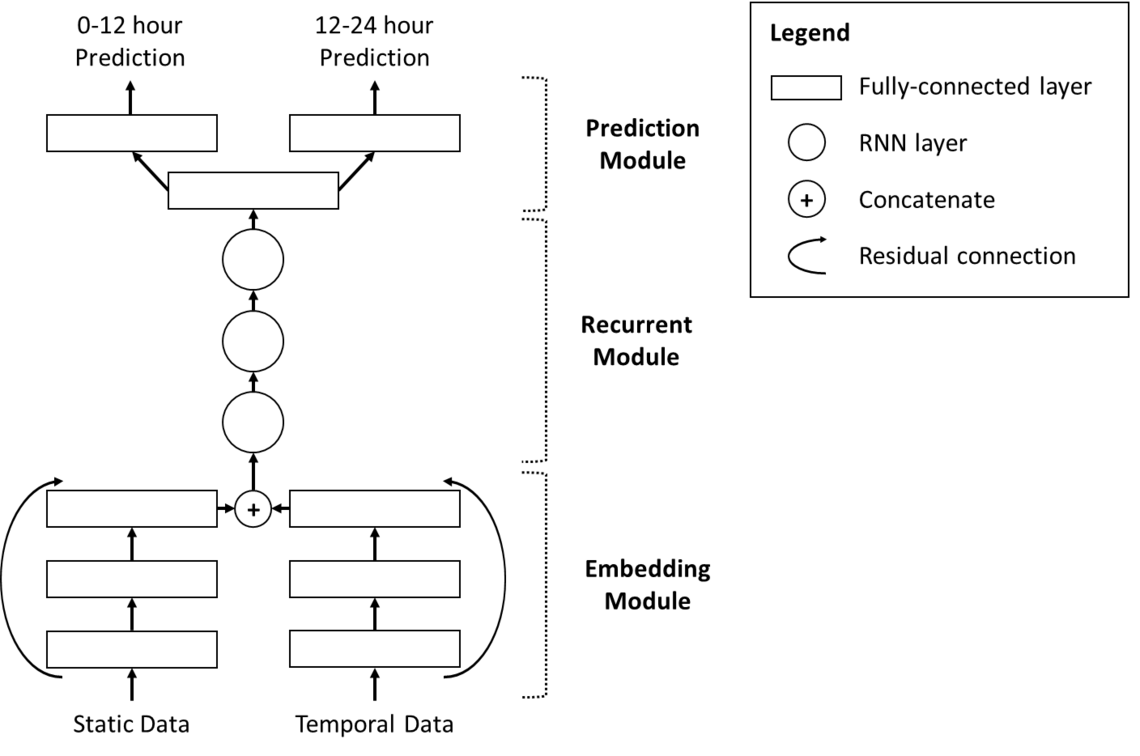
F. R. Lucini, H. T. Stelfox, and J. Lee. Deep Learning–Based Recurrent Delirium Prediction in Critically Ill Patients. Critical Care Medicine, 51(4), 492-502, April 2023. [GitHub]
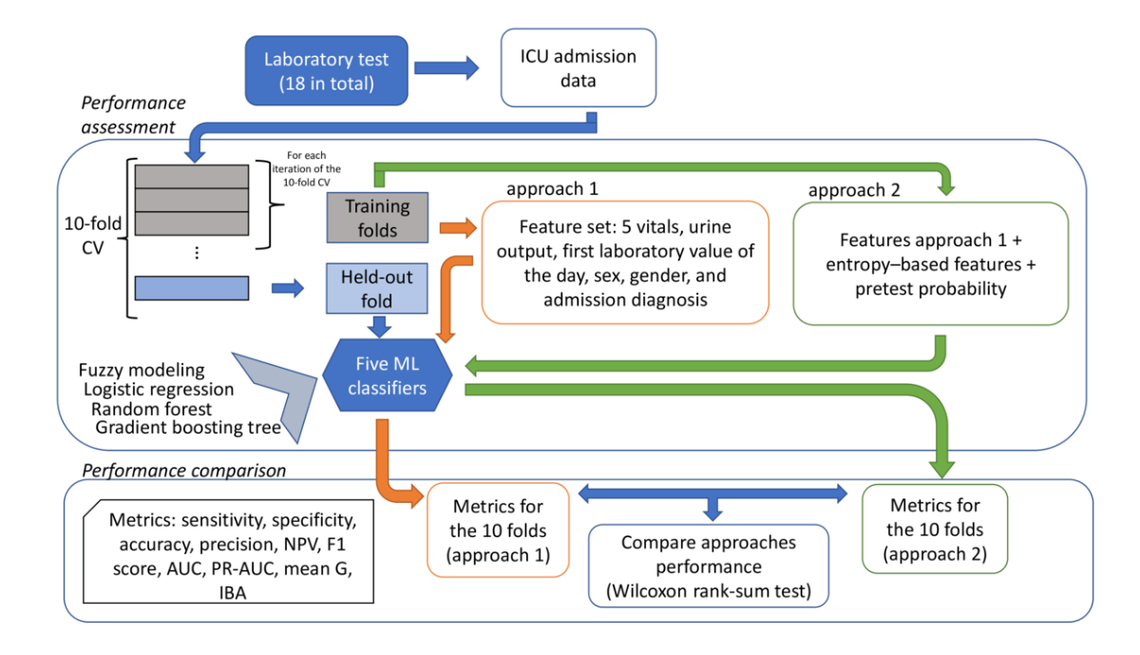
C. E. Valderrama, D. J. Niven, H. T. Stelfox, and J. Lee. Predicting abnormal laboratory blood test results in the intensive care unit using novel features based on information theory and historical conditional probability: observational study. JMIR Medical Informatics, 10(6):e35250, June 2022. [GitHub]
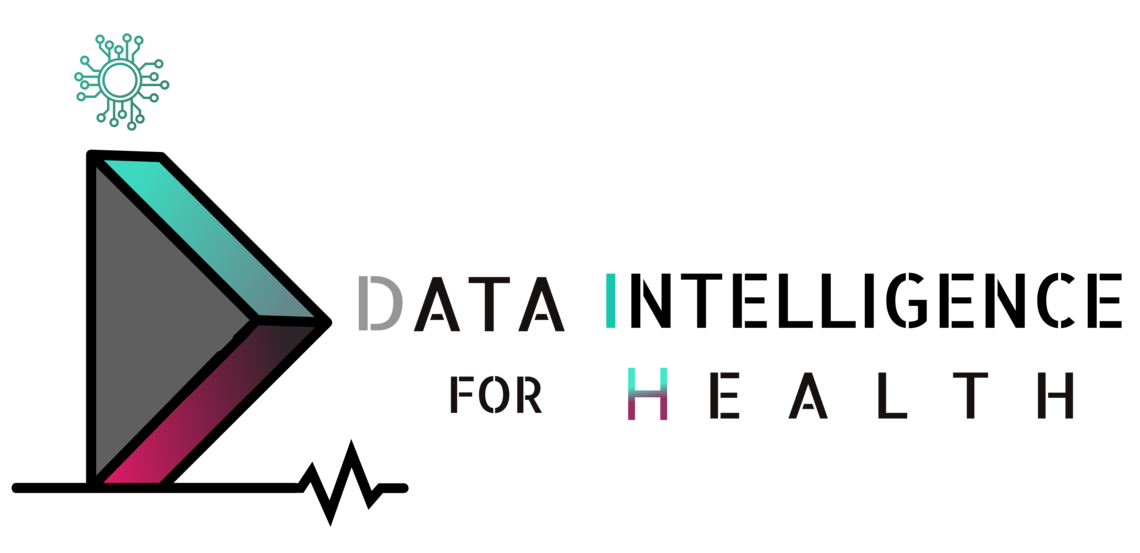
The ICU Family Presence Investigators. Family presence in adult intensive care units. Intensive Care Medicine, 48:759–761, May 2022.
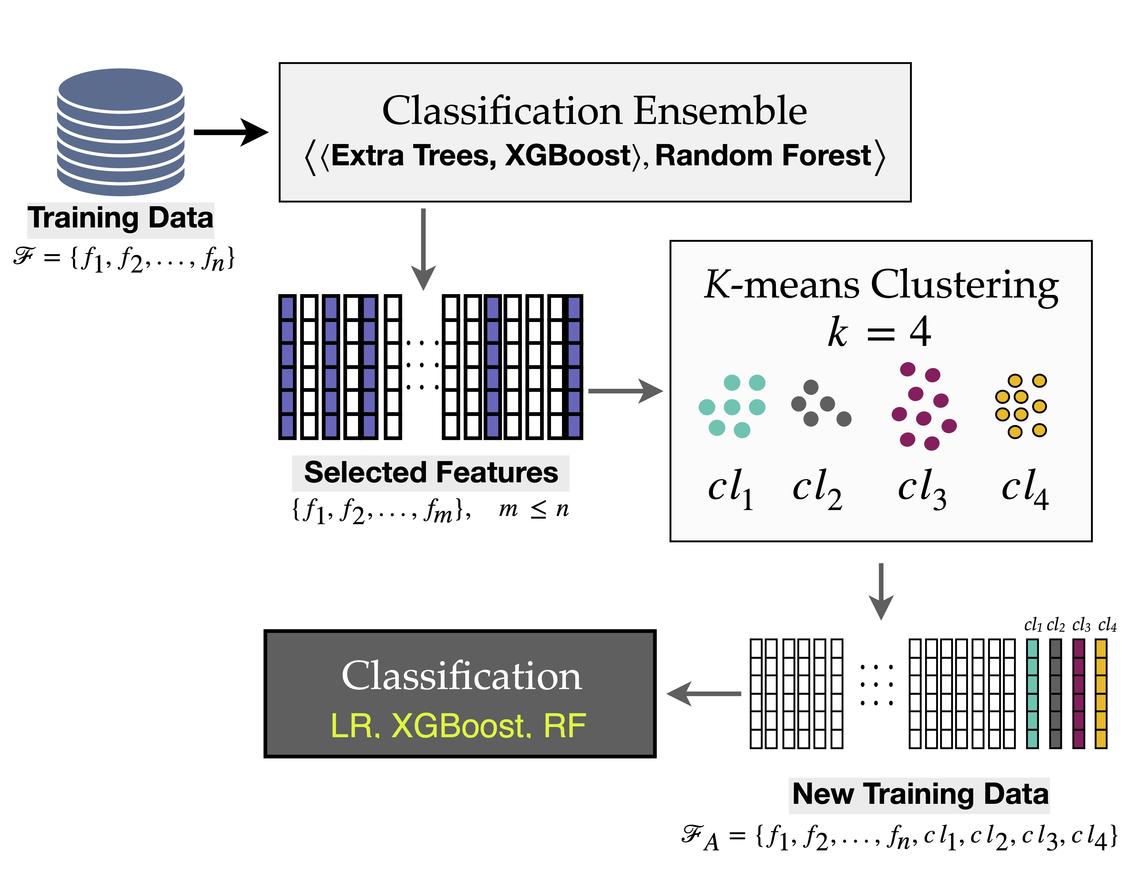
Z. Shakeri Hossein Abad, D. M. Maslove, and J. Lee. Predicting discharge destination of critically ill patients using machine learning. IEEE Journal of Biomedical and Health Informatics, 25(3):827-837, March 2021. [GitHub]
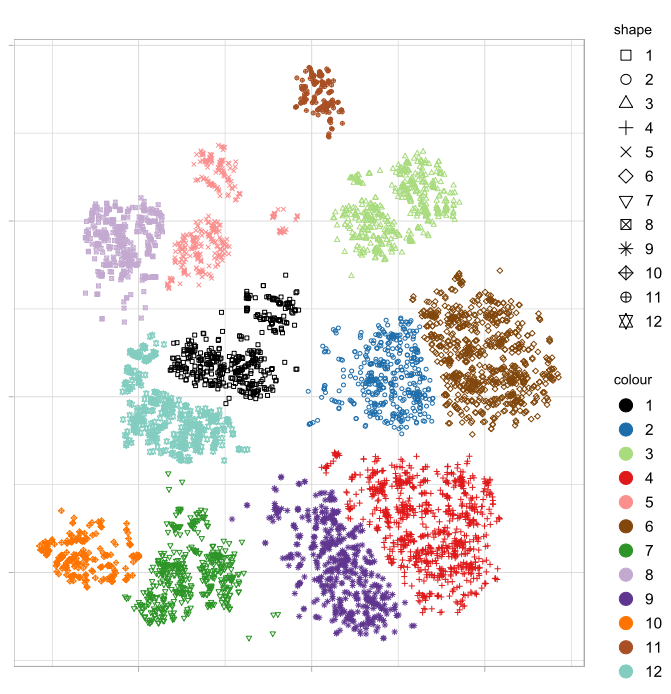
A. Sharafoddini, J. A. Dubin, and J. Lee. Identifying subpopulations of septic patients: a temporal data-driven approach. Computers in Biology and Medicine. 130:104182, March 2021.
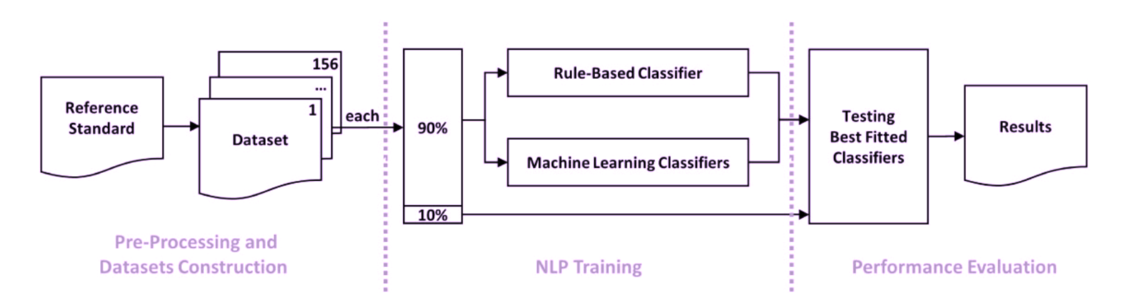
F. Lucini, K. D. Krewulak, K. M. Fiest, S. M. Bagshaw, D. J. Zuege, J. Lee, and H. T. Stelfox. Natural language processing to measure the frequency and mode of communication between healthcare professionals and family members of critically ill patients. Journal of the American Medical Informatics Association, 28(3):541-548, March 2021. [GitHub]
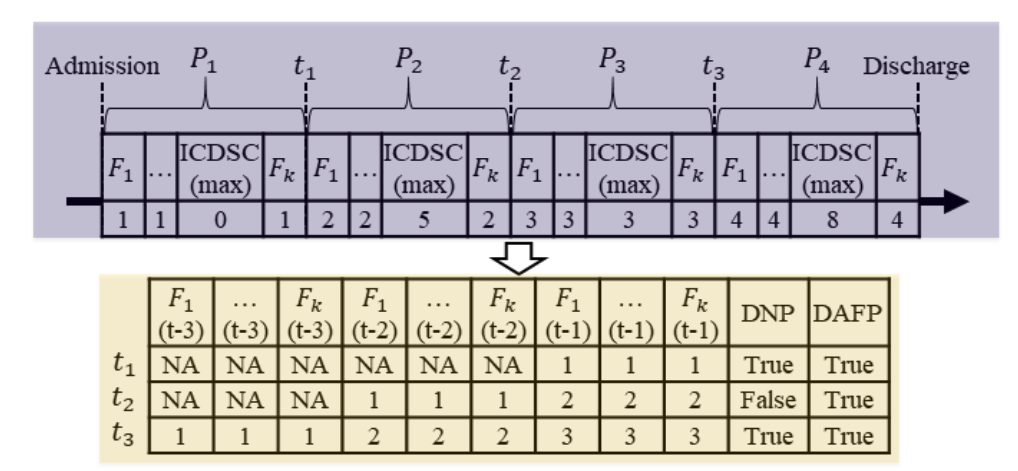
F. Lucini, K. Fiest, H. T. Stelfox, and J. Lee. Delirium prediction in the intensive care unit: a temporal approach. The 2020 Annual International Conference of the IEEE Engineering in Medicine and Biology Society, 5527-5530, 2020.
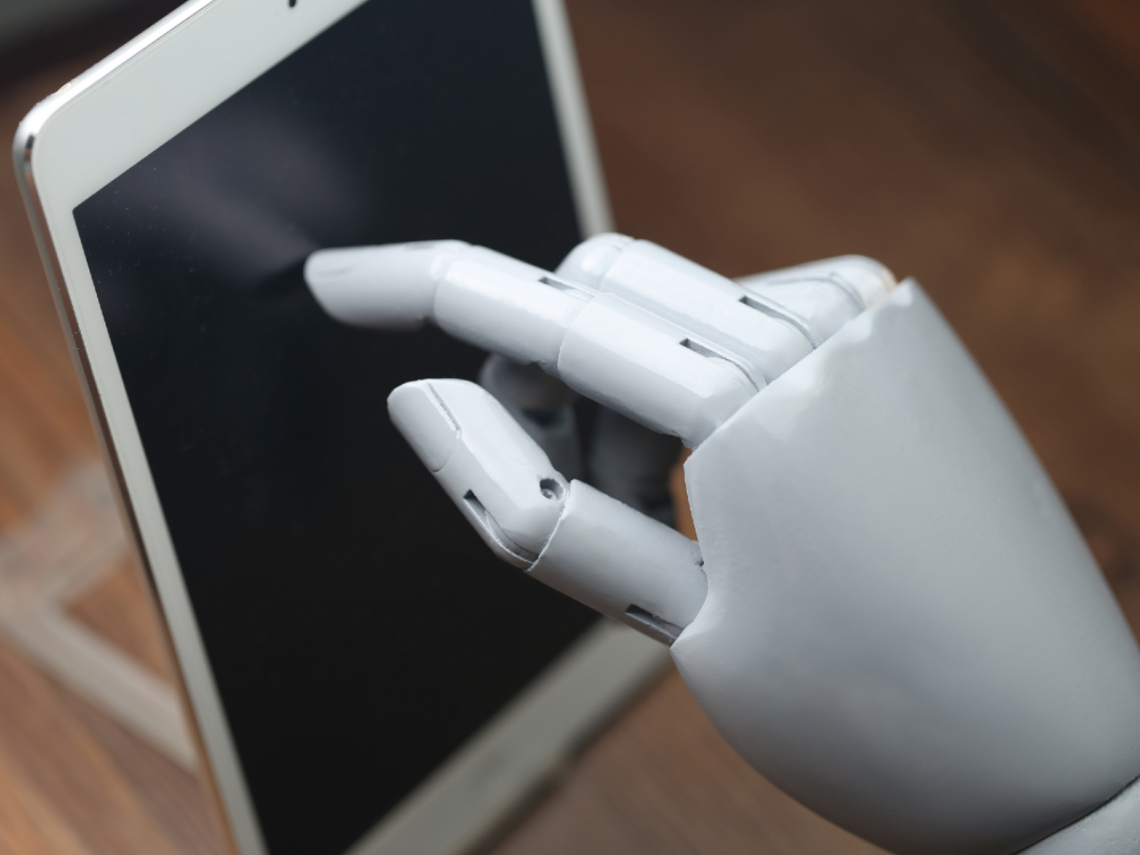
J. Lee. Is artificial intelligence better than human clinicians in predicting patient outcomes? Journal of Medical Internet Research, 22(8):e19918, August 2020.
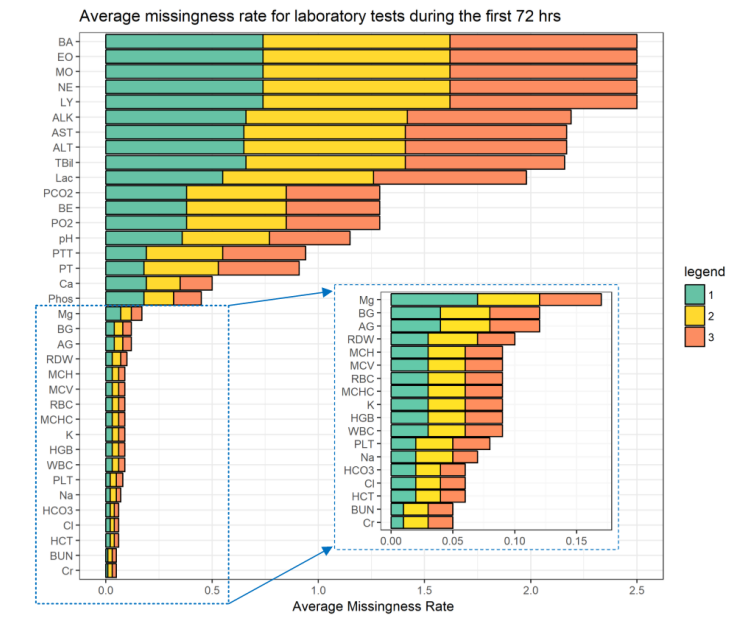
A. Sharafoddini, JA. Dubin, DM. Maslove, and J. Lee. A new insight into missing data in intensive care unit patient profiles: observational study, JMIR Med Inform 2019;7(1):e11605, January 2019.
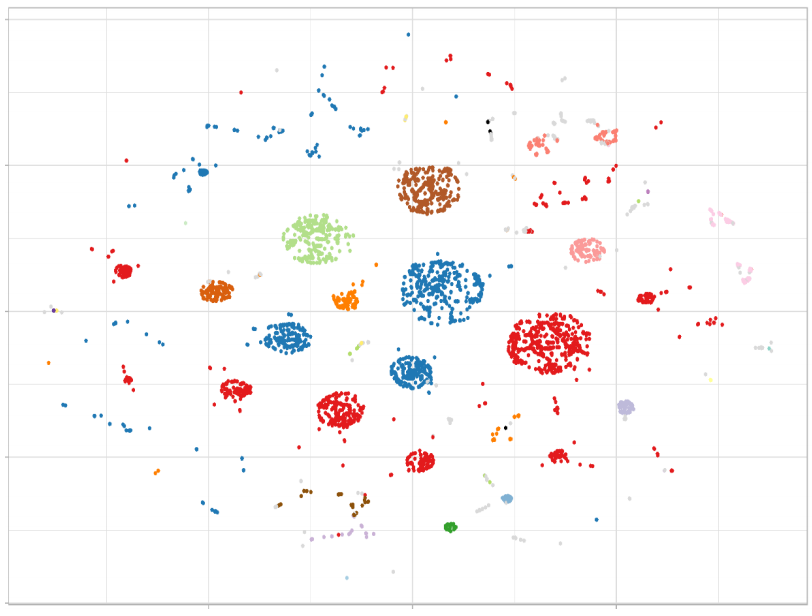
A. Sharafoddini, J. A. Dubin, and J. Lee. Finding similar patient subpopulations in the ICU using laboratory test ordering patterns. Proceedings of the 2018 7th International Conference on Bioinformatics and Biomedical Science (ICBBS), 72-77, 2018.
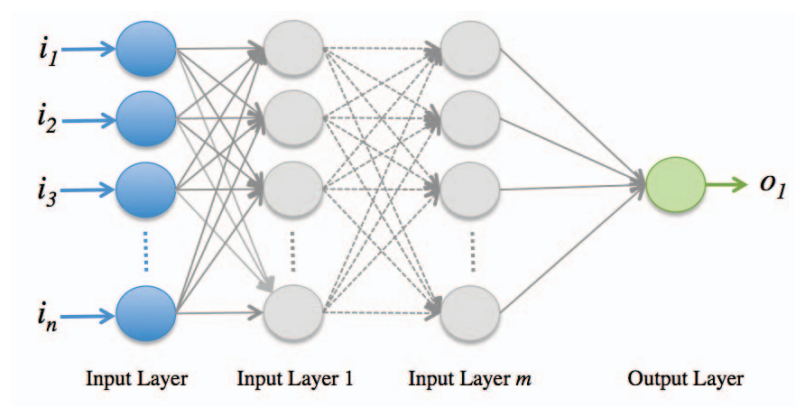
M. A. H. Zahid and J. Lee. Mortality prediction with self normalizing neural networks in intensive care unit patients. Proceedings of the 2018 IEEE-EMBS International Conference on Biomedical and Health Informatics, 226-229, 2018.
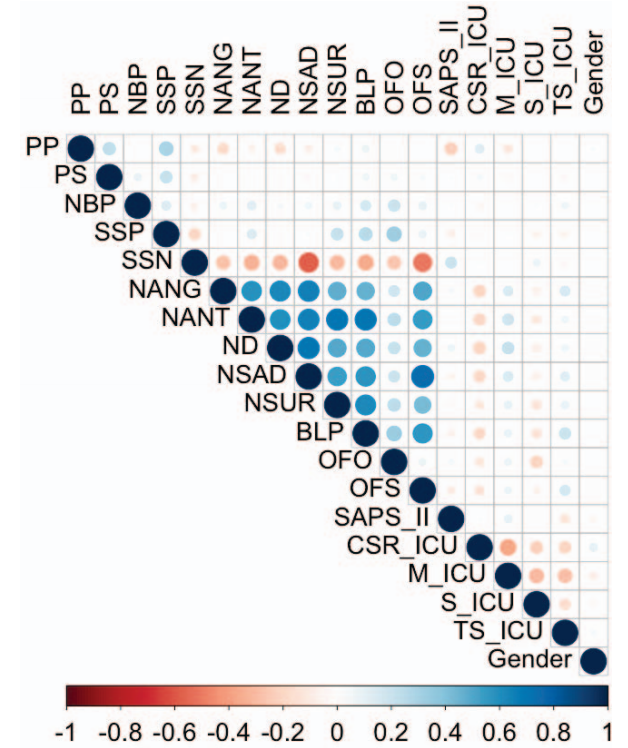
N. Tran and J. Lee. Using multiple sentiment dimensions of nursing notes to predict mortality in the intensive care unit. Proceedings of the 2018 IEEE-EMBS International Conference on Biomedical and Health Informatics, 283-286, 2018.
I. E. R. Waudby-Smith, N. Tran, J. A. Dubin, and J. Lee. Sentiment in nursing notes as an indicator of out-of-hospital mortality in intensive care patients. PLoS One, 13(6):e0198687, June 2018.
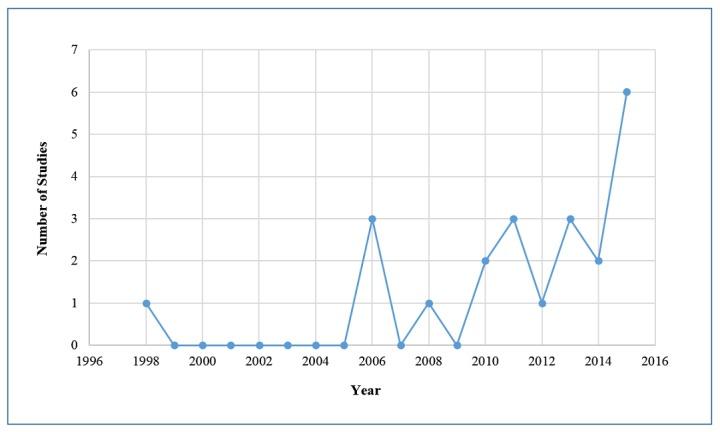
A. Sharafoddini, J. A. Dubin, and J. Lee. Patient similarity in prediction models based on health data: a scoping review. JMIR Medical Informatics, 5(1):e7, March 2017.
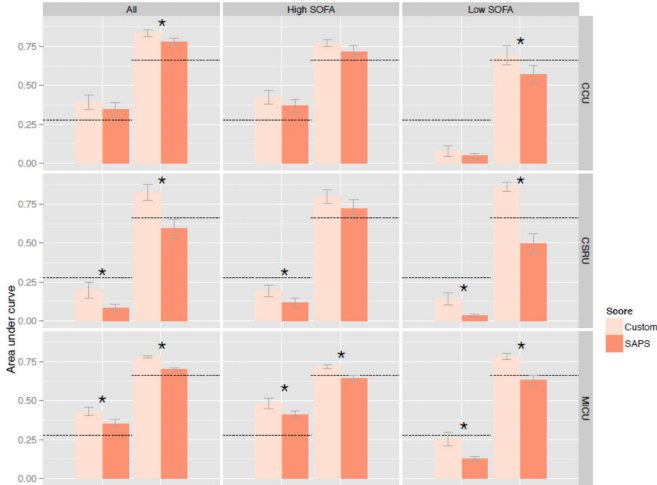
J. Lee and D. M. Maslove. Customization of a severity of illness score using local electronic medical record data. Journal of Intensive Care Medicine, 32(1):38-47, January 2017.
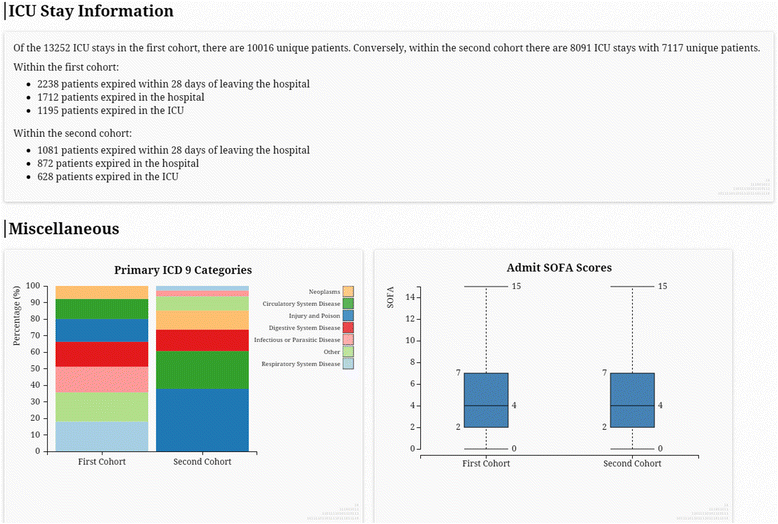
J. Lee, E. Ribey, and J. R. Wallace. A web-based data visualization tool for the MIMIC-II database. BMC Medical Informatics and Decision Making, 16:15, February 2016.
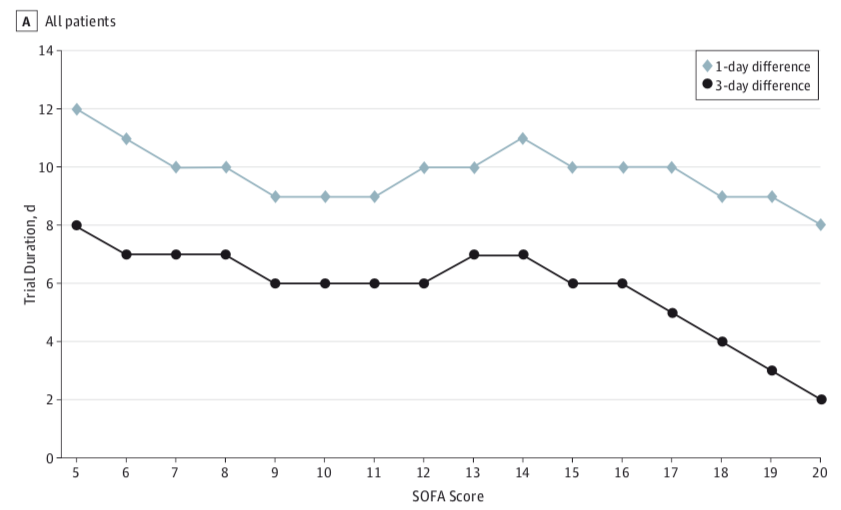
M. G. Shrime, B. S. Ferket, D. J. Scott, J. Lee, D. Bradford, T. Pollard, Y. M. Arabi, H. M. Al-Dorzi, R. M. Baron, M. G. Myriam Hunink, L. A. Celi, and P. S. Lai. How long is long enough? Time-limited trials for critically-ill patients with cancer. JAMA Oncology, 2(1):76-83, January 2016.
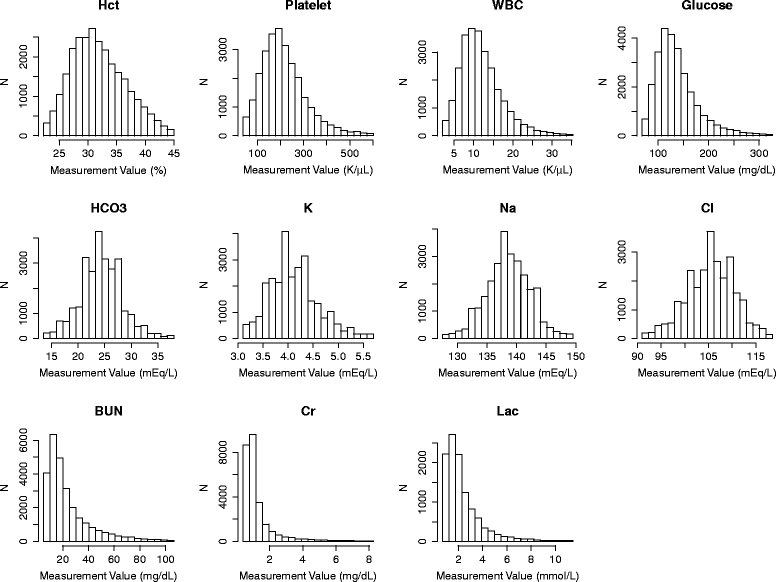
J. Lee and D. M. Maslove. Using information theory to identify redundancy in common laboratory tests. BMC Medical Informatics and Decision Making, 15:59, July 2015.
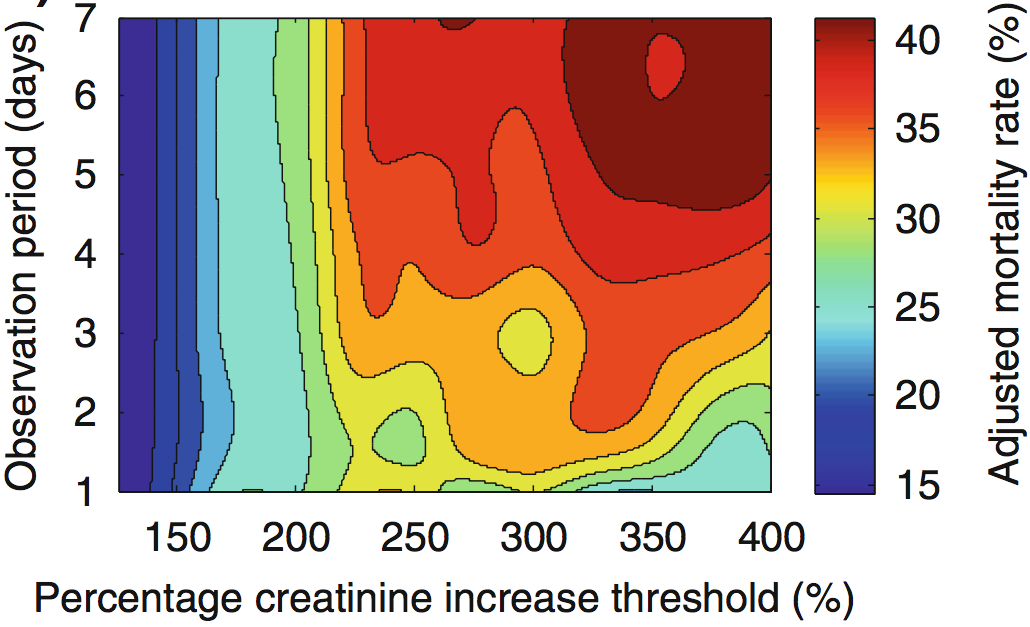
T. Mandelbaum, J. Lee, D. J. Scott, R. G. Mark, A. Malhotra, M. D. Howell, and D. Talmor. Empirical relationships among oliguria, creatinine, mortality, and renal replacement therapy in the critically ill. Intensive Care Medicine, 39(3):414-419, March 2013.
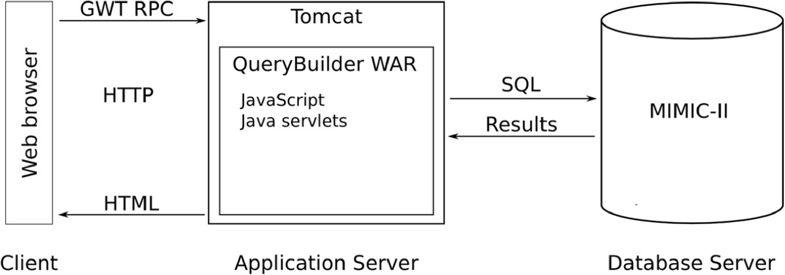
D. J. Scott, J. Lee, I. Silva, S. Park, G. B. Moody, L. A. Celi, and R. G. Mark. Accessing the public MIMIC-II intensive care relational database for clinical research. BMC Medical Informatics and Decision Making, 13:9, January 2013.
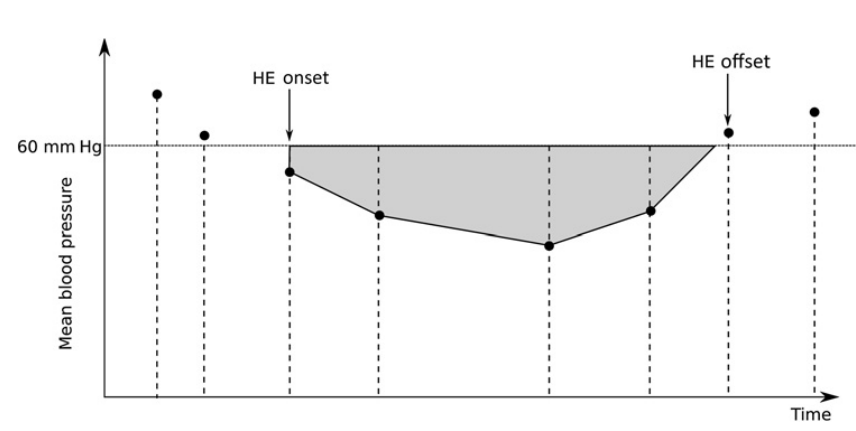
J. Lee, R. Kothari, J. A. Ladapo, D. J. Scott, and L. A. Celi. Interrogating a clinical database to study treatment of hypotension in the critically ill. BMJ Open, 2(3):e000916, June 2012.
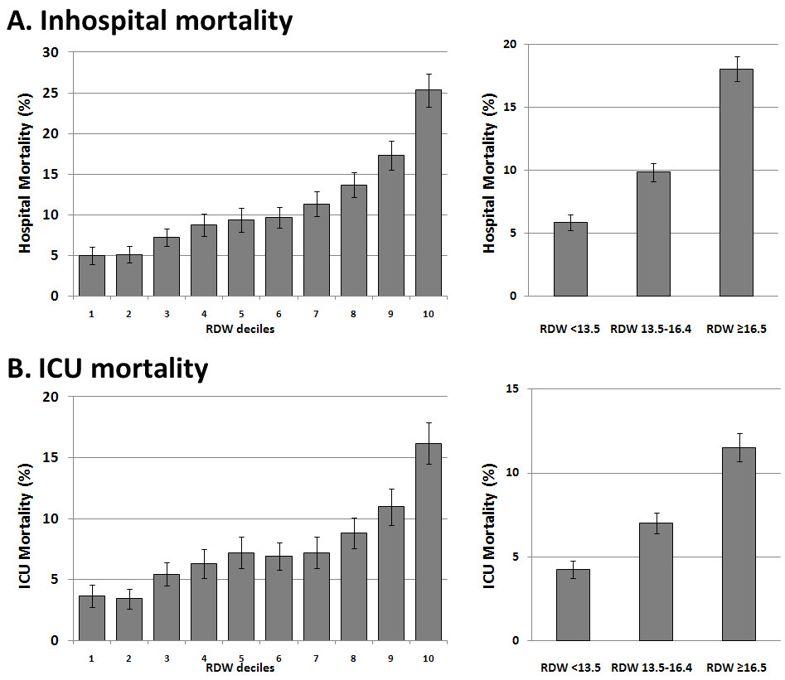
S. Hunziker, L. Celi, J. Lee, and M. D. Howell. Red cell distribution width improves the SAPS score for risk prediction in unselected critically ill patients. Critical Care, 16(3):R89, May 2012.
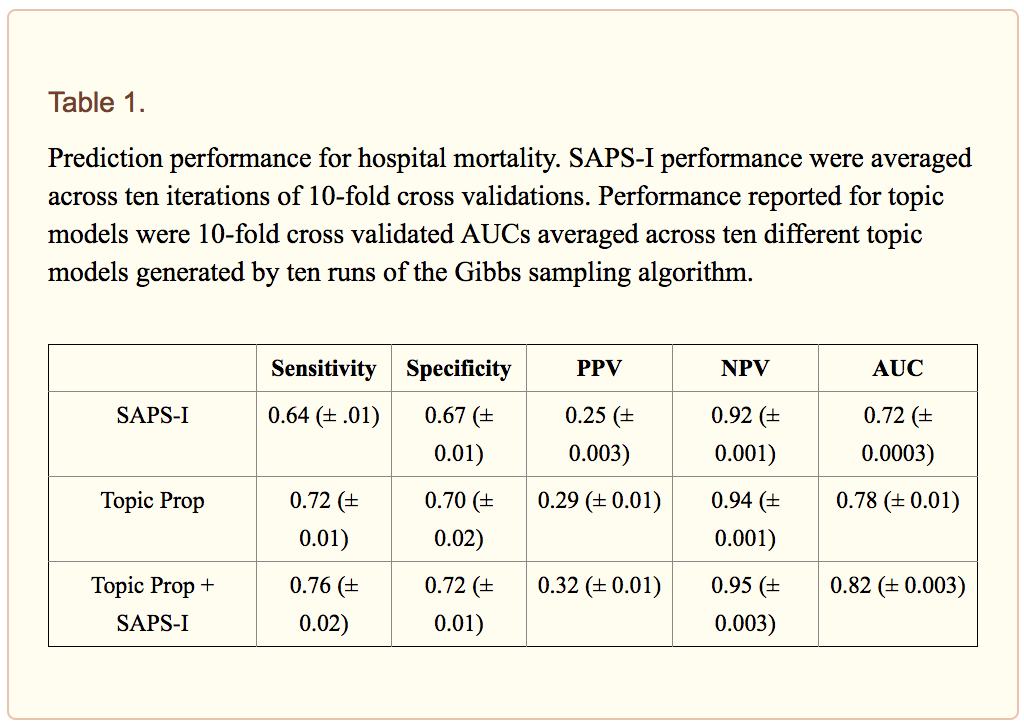
L. Lehman, M. Saeed, W. Long, J. Lee, and R. Mark. Risk stratification of ICU patients using topic models inferred from unstructured progress notes. Proceedings of the American Medical Informatics Association 2012 Annual Symposium, 505-511, 2012.
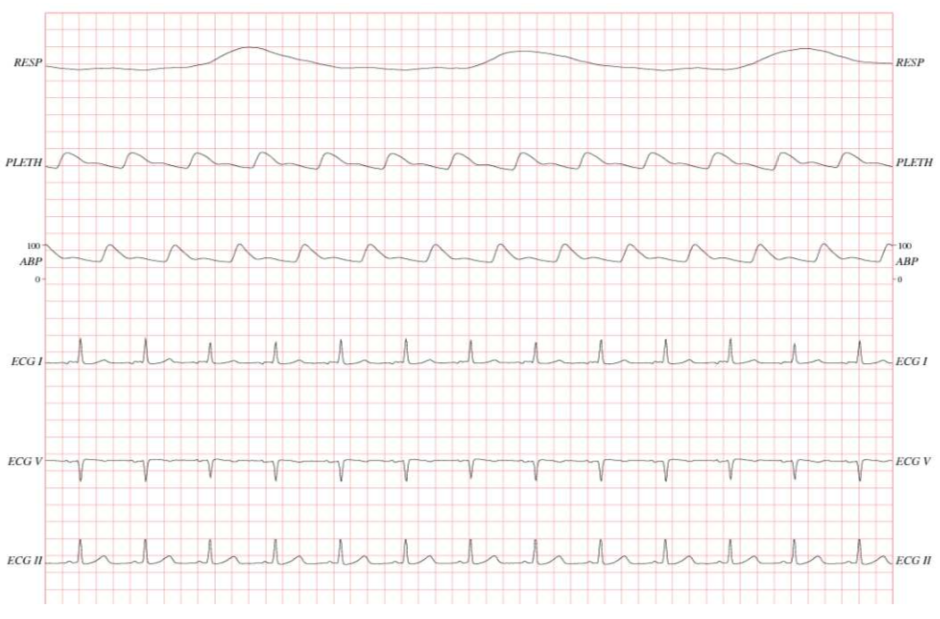
J. Lee, D. J. Scott, M. Villarroel, G. D. Clifford, M. Saeed, and R. G. Mark. Open-access MIMIC-II database for intensive care research. Proceedings of the 33rd Annual International Conference of the IEEE Engineering in Medicine and Biology Society, 8315-8318, 2011.
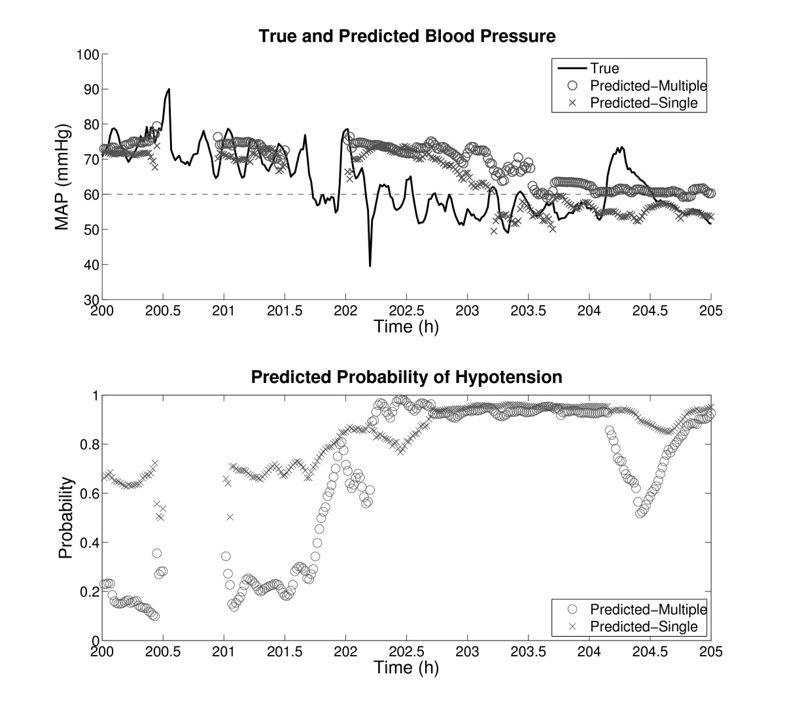
J. Lee and R. G. Mark. An investigation of patterns in hemodynamic data indicative of impending hypotension in intensive care. BioMedical Engineering OnLine, 9:62, October 2010.
Funded By
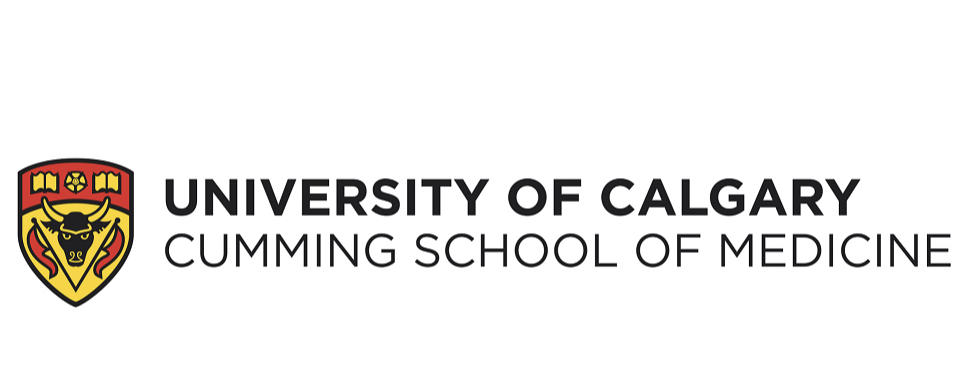
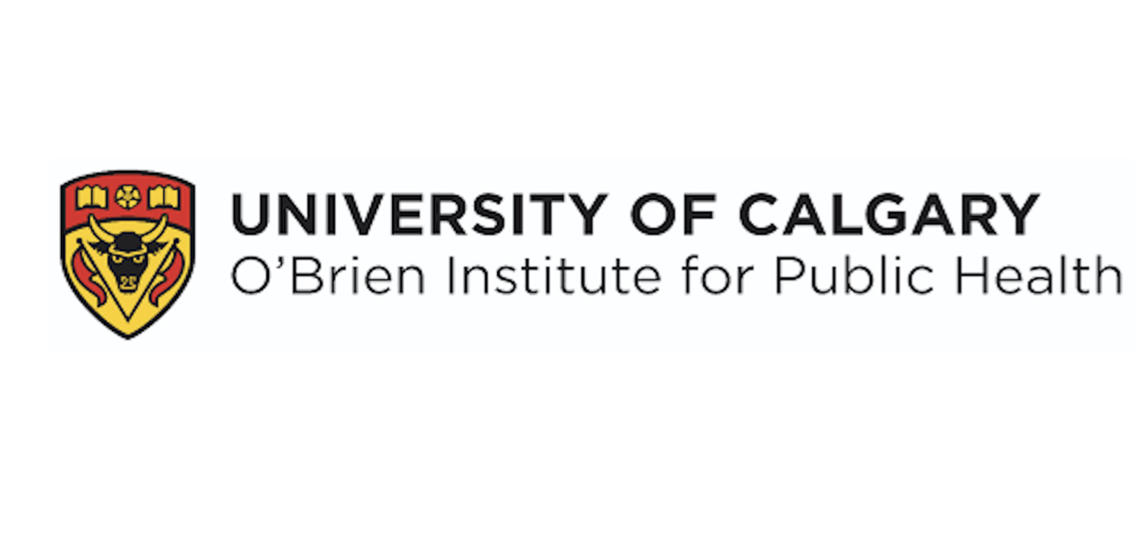
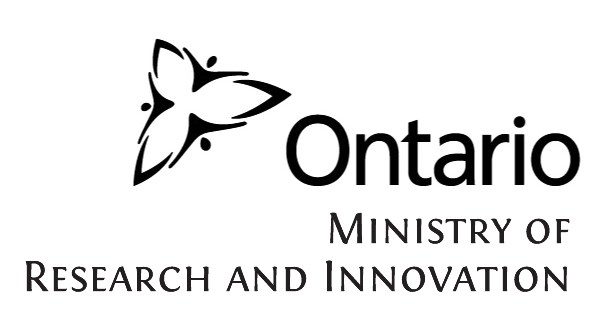
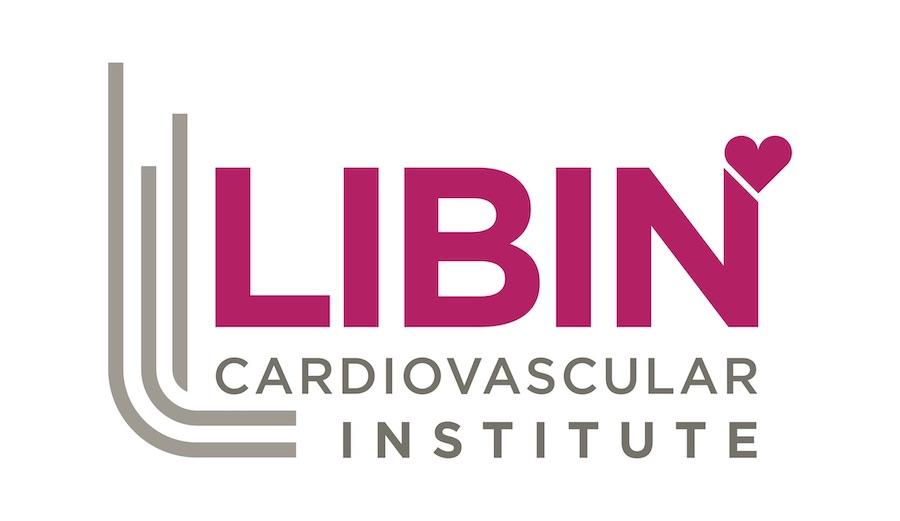
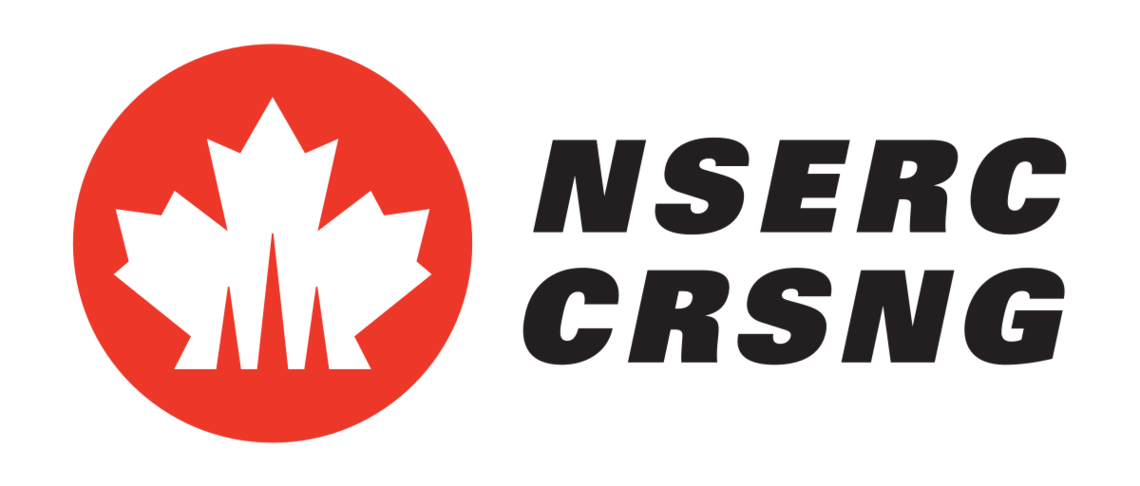