Deep Learning-based Image Reconstruction
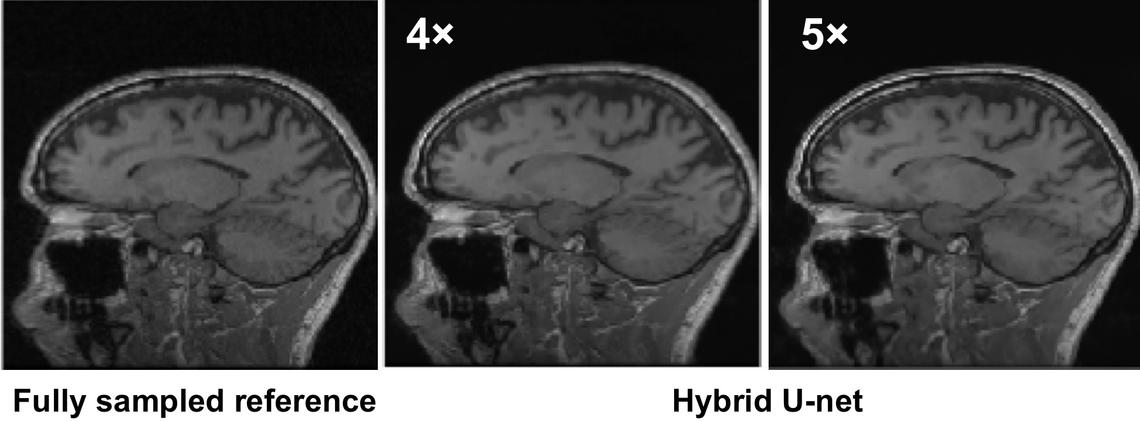
Example of deep-learning reconstructed images from undersampled data (R=4 and 5) versus the fully sampled reference image.
This research investigates the application of deep learning networks for reconstruction of undersampled MR datasets.
The work extends our extensive experience with compressed sensing (CS) and projection-on-to-convex sets (POCS) strategies over 2005-2018. Our application of deep learning approaches is widespread and includes applications in phased-array coil imaging and brain perfusion/permeability imaging, as well as medical image decompression.
Since 2018, we have been exploring the application of deep-learning based methods for MR image reconstruction and introduced the pioneering hybrid-domain W-net concept.
This research activity is principally funded by NSERC and occurs in collaboration with Roberto Souza from Electrical and Software Engineering.
Representative Publications
- Yerly J, Lauzon ML, Chen HS, Frayne R. A simulation-based analysis of the potential of compressed sensing for accelerating passive MR catheter visualization in endovascular therapy. Magn Reson Med. 2010; 63: 473-83.
- Sabati M, Peng H, Lauzon ML, Frayne R. A statistical method for characterizing the noise in nonlinearly reconstructed images from undersampled MR data: The POCS example. Magn Reson Imaging 2013; 31: 1587-98. doi: 10.1016/j.mri.2013.06.011
- Souza R, Bento M, Nogovitsyn N, Chung KJ, Loos W, Lebel RM, Frayne R. Dual-domain cascade of U-nets for multi-channel magnetic resonance image reconstruction. Magn Reson Imaging. 2020; 71: 140-153. doi: 10.1016/j.mri.2020.06.002.
- Souza R, Beauferris Y, Loos W, Lebel RM, Frayne R. Enhanced deep-learning-based magnetic resonance image reconstruction by leveraging prior subject-specific brain imaging: Proof-of-concept using a cohort of presumed normal subjects. IEEE Journal of Selected Topics in Signal Processing 2020; 14: 1126-1136. doi: 10.1109/JSTSP.2020.3001525.
- Beauferris Y, Teuwen J, Karkalousos D, ..., Loos W, Frayne R, Souza R. Multi-coil MRI reconstruction challenge–assessing brain MRI reconstruction models and their generalizability to varying coil configurations. Frontiers in Neuroscience 2022; 16: 919186. doi: 10.3389/fnins.2022.919186.
Prospective Trainee Requirements
Interest in image reconstruction and deep learning with a degree (MSc preferred) in Biomedical, Computer, Electrical, or Software Engineering. A good grasp of digital signal processing techniques, statistics, and/or experience with numerical optimization methods would be highly beneficial. Must have good oral and written English communication skills.