Management of Study Variability/Quality Control
The research focuses on the quality assessment and proper management of large clinical imaging studies and trials.
By indentifying and understanding data points that belong (inliers) and do not belong (outliers) to a dataset, the overall accuracy and precision of a processing method can be improved. A major focus of our work is on the application of novel machine learning approaches to help decide what is "good" data, and understanding and mitigating of bias in our studies.
The work is in conjunction with Mariana Bento from Biomedical Engineering, and is supported by the Calgary Image Processing and Analysis Centre (CIPAC).
Representative Publications
- METACOHORTS Consortium: Dichgans M, Wardlaw J, Smith E, Zietemann V, et al., METACOHORTS for the study of vascular disease and its contribution to cognitive decline and neurodegeneration: An initiative of the Joint Programme for Neurodegenerative Disease Research. Alzheimers Dement. 2016; 12: 1235-1249. doi: 10.1016/j.jalz.2016.06.004.
- Bento M, Souza R, Frayne R. Multicenter Imaging Studies: Automated Approach to Evaluating Data Variability and the Role of Outliers. 31st SIBGRAPI Conference on Graphics, Patterns and Images (SIBGRAPI) 2018: 182-188.
- Smith EE, Biessels GJ, De Guio F, de Leeuw FE, Duchesne S, Düring M, Frayne R, et al. Harmonizing brain magnetic resonance imaging methods for vascular contributions to neurodegeneration. Alzheimers Dement (Amst). 2019; 11: 191-204. doi: 10.1016/j.dadm.2019.01.002.
- Bento M, Fantini I, Park J, Rittner L, Frayne R. Deep Learning in Large and Multi-Site Structural Brain MR Imaging Datasets. Frontiers in Neuroinformatics 2022; 15. doi.org/10.3389/fninf.2021.805669.
Prospective Trainee Requirements
MSc or PhD in Mathematics, Computer Science, Electrical or Computer Engineering, Physics or a closely aligned field. Good skills and experience in statistics, and image processing and analysis techniques. Understanding of modern software engineering principles. Experience in C++ or Python or similar language and pattern recognition techniques is desired. Good written and oral English language skills.
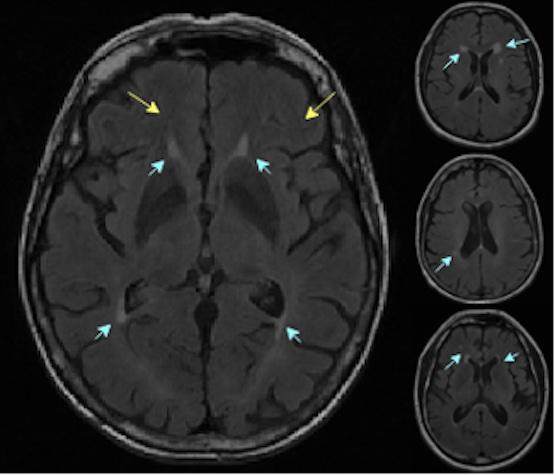
Automated identification of white matter hyperintensities on MR imaging.