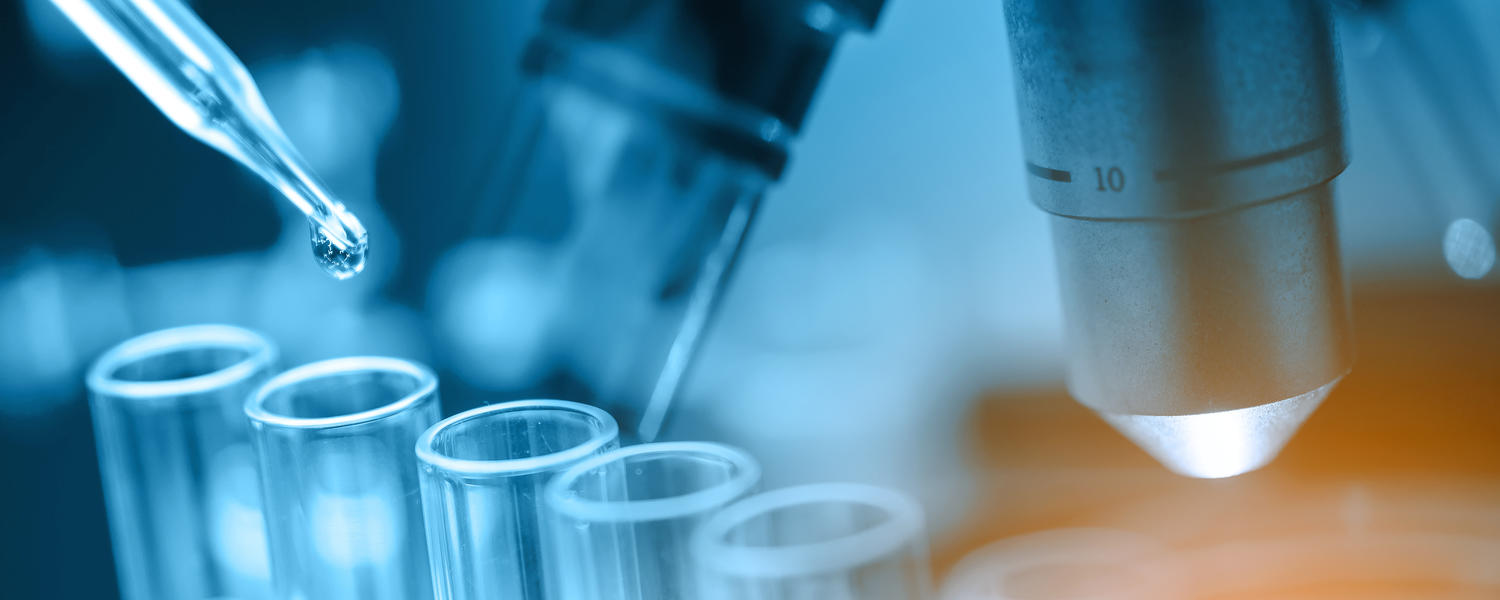
Office of Surgical Research | Statistical Support
Statistical Support
The Office of Surgical Research (OSR) provides statistical support to all Department of Surgery members, residents, and medical students supervised within the department. We ask anyone who requests biostatistical support to complete a short survey. Completion of the survey allows us to triage requests and help determine the level of support required. We will contact you after your submission is received and reviewed.
If you require more information or have questions, please contact our biostatistician.
Why Should a Statistician be Involved in Research Design?
There are many benefits to involving a statistician in the process of research design. The statistician can help define and develop your research question, create surveys and questionnaires, determine the optimal sample size, reduce sampling bias, and help create a well-formatted dataset. The statistician will work with you towards the successful completion of the project.
The following information is provided to help guide researchers through sample size calculations, data collection, and data set submissions. Please note that this information is provided only as a guide and does not replace proper consultation with the biostatistician.
Sample Size Calculation
These questions will be asked when a sample size calculation request is received. The statistician will clarify the questions during your first meeting, but we suggest reviewing them before proceeding with data collection.
- What is your desired level of significance? Most researchers use 95% or higher.
- What is your desired power? Most researchers use 80% or higher. Learn more about statistical power.
- What test are you going to use? The test you use depends on the type of dependent and independent variables you have. Consult with the statistician if you are not sure.
- What is your expected effect size? Learn more about effect size
- What percentage of patients do you estimate will drop out?
- What is the proportion of samples in your groups? Sometimes it is easier to get more samples from one group than another group, but this affects the required sample size.
Review the article Power Analysis & Sample Size Estimation to learn more about sample size calculations.
Data Collection and Submission
When collecting data and submitting your spreadsheets for statistical analysis, there are several things to consider. Following the below guide, although not required, may save significant time in the future. Fixing errors often requires several meetings with your statistician, and you may need to check the original documents. Sometimes finding the correct information is not even feasible. Once the issues are resolved, there is a risk of more than one 'original file,' which may create chaos - this happens quite often. The best practice is to think about your data collection and data formatting in advance.
General Guides
- Protect the identity of the patients (e.g., use IDs instead of patient names).
- Plan your spreadsheet.
- Do not leave blank rows or columns in the middle of a dataset.
- Start data entry from column A. Do not include rows with the project title.
- Typically, each variable is entered in a column, and each subject is entered in a row.
- Avoid duplicate subjects. If you must, add extra columns.
- The first row should be column names.
- Avoid double headers (more than one row with column names).
- Avoid merging cells.
- Avoid colour-coding the rows. You can usually add a new column and define your groups in a separate column.
- Do not use charts/graphs in your datasheet.
- Group similar items in the same column. Use the same units of measurement in each column.
Guides for Column Names
- All columns must have a name.
- Avoid very long column names.
- Avoid using symbols in column names (i.e., spaces, -, parentheses, %, @, &, $). Underscore is okay (e.g., use BloodPressure or Blood_Pressure instead of Blood Pressure or Blood-Pressure)
- Do not start column names with numbers.
- Avoid duplicate column names, even if they differ in upper/lower case. If you have two columns with the same name, check for matching entries before you delete a column.
Guides for Data Entry
- All values in any given column must have a similar format.
- Any character added to a number will make it a “string” or “text.” For example, if you have a column for height, all values should be numbers and use the same units. It is not acceptable to use 130cm, ?, <150, or 5’ 6” because these are read as “strings” or "text."
- Be consistent in data entry. Use any of M, m, 1, Male, male, Man, Men, Male (note: spaces are hard to spot), but be consistent.
- Beware of dates! You can write 01/06/14 and 06/01/14 and have no idea which number is the day, month, or year until you later when serious errors are found (e.g., negative survival times). It is best to record dates with the following format 18 May 2021.
- Do not include a statistical calculation such as a mean or standard deviation in the original data.
- Check your numbers to ensure they make sense (e.g., Patient age: 230 years).
- Leaving cells blank almost always raises questions of whether data is missing or not applicable. Write as “unknown” if missing and “NA” if not applicable.
Merging Different Spreadsheets
To properly merge two spreadsheets, you need to have an index column with unique values (i.e., no duplicate values) that is shared in both spreadsheets.
Watch Your Missing Data
Missing data can occur in almost any dataset. They can be produced during research design or during data collection. In the statistical analysis of data, it is important to understand the nature of missing data and to deal with it accordingly. This ensures we will minimize bias and increase statistical power. There are three main types of missing data:
- Missing completely at random (MCAR) is when the probability of missing data is not related to any other parameter and data is missing by pure chance.
- Missing at random (MAR) is when there is a reason behind missingness that can be identified through other observed variables. For example, a geologist is not sampling a specific unit in a gold exploration project because he is certain that there is no gold associated with that geologic unit.
- Missing not at random (MNAR) is when missingness depends on information that has not been recorded. For example, a certain cancer is associated with smoking, but the data on whether or not patients smoked is not recorded.
There are two main mechanisms for dealing with missing data:
- Discard the missing values
- Impute the missing values
Discarding data is an easy approach but should be used with caution and under certain conditions. If a large percentage of data is missing, discarding it results in reducing statistical power. Also, if there is a reason behind missingness, discarding it results in bias. For example, if males are less likely to answer questions about depression status compared to females, then discarding missing values will introduce bias.
Missing data can be imputed through simple approaches like mean imputation, last value carried forward, using information from related observations, or based on logical rules. These methods need to be used with sound judgment. For example, it makes sense to impute a missing temperature from an hour before, but it does not make sense to impute someone’s blood pressure based on a previous patient’s blood pressure. Also, it is important to note that these methods can reduce standard error of estimates toward zero.
The more complicated techniques to impute data include random imputation, regression, random regression, matching and hot-deck imputations, as well as multiple imputations.
Reference: http://www.stat.columbia.edu/~gelman/arm/missing.pdf
Choosing a Statistical Test
The correct statistical test depends on your study design and the characteristics of your data. Remember that each statistical test must satisfy its underlying “assumptions” to be valid. For example, with comparison and association tests, it is often important to verify whether or not the data is drawn from a population that is normally distributed. The parametric tests are tests that assume population data are normally distributed. Non-parametric tests are independent of underlying data distributions and should be used for non-normal variables.
In determining the correct statistical test, it is important to clearly define the goal of the study, determine dependent variables or independent variables, types of variables: continuous (quantitative), categorical (ordinal), categorical (nominal), or categorical (binary), and determine the outcome variable population distribution. It is also important to decide whether your groups are paired (e.g., comparing patients' blood sugars before and after surgery) or independent (e.g., comparing control versus intervention groups).
The following tables are provided as a guide when choosing the statistical test for your project. The non-parametric equivalents for tests of comparison and association are provided in parentheses. View the PDF.